Advice for CIOs: How to Build IT Architecture for Diverse New Applications
Diverse data applications such as distributed databases, big data analytics, high-performance computing (HPC), and AI are booming.
Over three decades, data storage has evolved to become the optimal foundation of high-value data in line with the development of new data applications as industries go digital. As well as traditional database applications, distributed databases, big data, and AI applications are all emerging.
According to statistics, each enterprise has more than 100 types of data applications.
Immature IT stacks for new data applications need tiering standards
When new data applications emerged in the past, integrated server architecture with coupled applications and local disks was often used to rapidly deploy innovative services. However, the gap between the computing power lifecycle and data lifecycle is rapidly increasing as these services become production services. Computing and storage resources need to be planned and maintained flexibly and independently. Enterprises begin to focus on reliability and cost-effectiveness. The architecture based on the local disks of servers is far less reliable than external storage. In addition, compute and storage cannot be independently expanded, resulting in high hardware costs, idle resources, and low utilization.
Distributed databases
At the early stage of its emergence, enterprises adopted the resource-wasting coupled storage-compute IT stack architecture. Both compute and storage resources need capacity expansion, when either are insufficient. As technologies become mature, distributed databases, such as Amazon Aurora, run on a decoupled storage-compute architecture that makes IT resources more flexible to expand and saves more than one third in server resources. At the same time, enterprise-grade all-flash storage notably improves data reliability.
Data analytics
Big data analytics is essential for guiding business operations. Since 2010, enterprises have turned to Hadoop. Its local data storage design creates a coupled storage-compute architecture. As data processing scales increase from TBs to PBs, the disadvantages of the coupled storage-compute architecture have gradually been exposed, specifically low resource utilization and high deployment costs.
Some enterprises are exploring IT architecture innovations. For example, one carrier replaced its coupled architecture with new decoupled storage-compute architecture.
The decoupled model slashes the hardware and software costs of cabinets, servers, and electricity by an average of 40% and annual carbon emissions by more than 50%.
Why joint innovation matters
As emerging applications such as big data and AI are widely used in enterprises, the efficiency of data applications determines an enterprise’s level of digitalization.
With the development and segmentation of new application scenarios, joint innovation of applications, compute, and storage is required to build efficient solutions for growing sub-scenarios.
For example, storage vendors are building acceleration engines as joint innovation carriers for diverse data applications.
Figure 1: Application acceleration for near-data processing
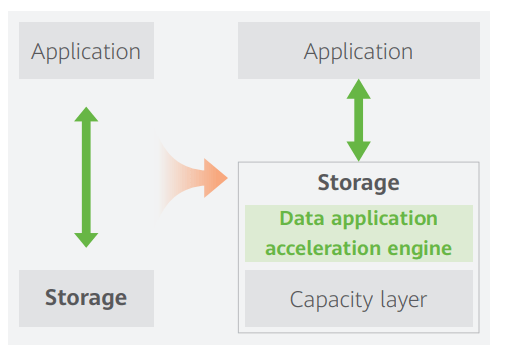
- Distributed database application acceleration engine
Currently, most mainstream distributed databases can only enable write-once and read-many, requiring database/table sharing. The database application acceleration engine can enable write-many and read-many for distributed databases, minimizing application reconstruction.
- Big data application acceleration engine
The data access latency of the traditional Hadoop big data platform is hundreds of microseconds and data analysis latency reaches days. A distributed storage high-speed cache built to move application operators to the storage layer reduces data access latency to 10 microseconds and accelerates big data analysis efficiency to minutes.
- HPDA application acceleration engine
High-performance data analytics involves mixed data types of large and small files, posing high requirements on bandwidth and OPS. Traditional storage devices can only support the access performance of a single data type. To resolve this, parallel data access clients that support cache acceleration are deployed at the compute layer, and metadata access is accelerated at the storage layer. This meets the high-performance requirements for mixed access of large and small files.
- AI application acceleration engine
AI training evolves to large and multimodal models, increasing the number of modeling parameters by 10 to 100 times. The AI acceleration engine accelerates the feature processing and intelligent scheduling of pipeline tasks, improving AI training efficiency by dozens of times, accelerating the training period, and controlling time costs.
What we recommend
- Use the decoupled storage compute architecture for emerging data applications to improve reliability and cost effectiveness
With digitalization deepening, diverse data applications are becoming new production applications that require much more from data reliability. As the gap between the computing power lifecycle and data lifecycle is rapidly growing, compute and storage resources need to be planned and maintained flexibly and independently.
It is recommended that elastic, reliable, and cost-effective professional storage running on a decoupled storage-compute architecture should be used for diverse data applications to rapidly deploy innovative services and improve reliability and cost-effectiveness.
Figure 2: Decoupled storage-compute architecture for emerging data applications
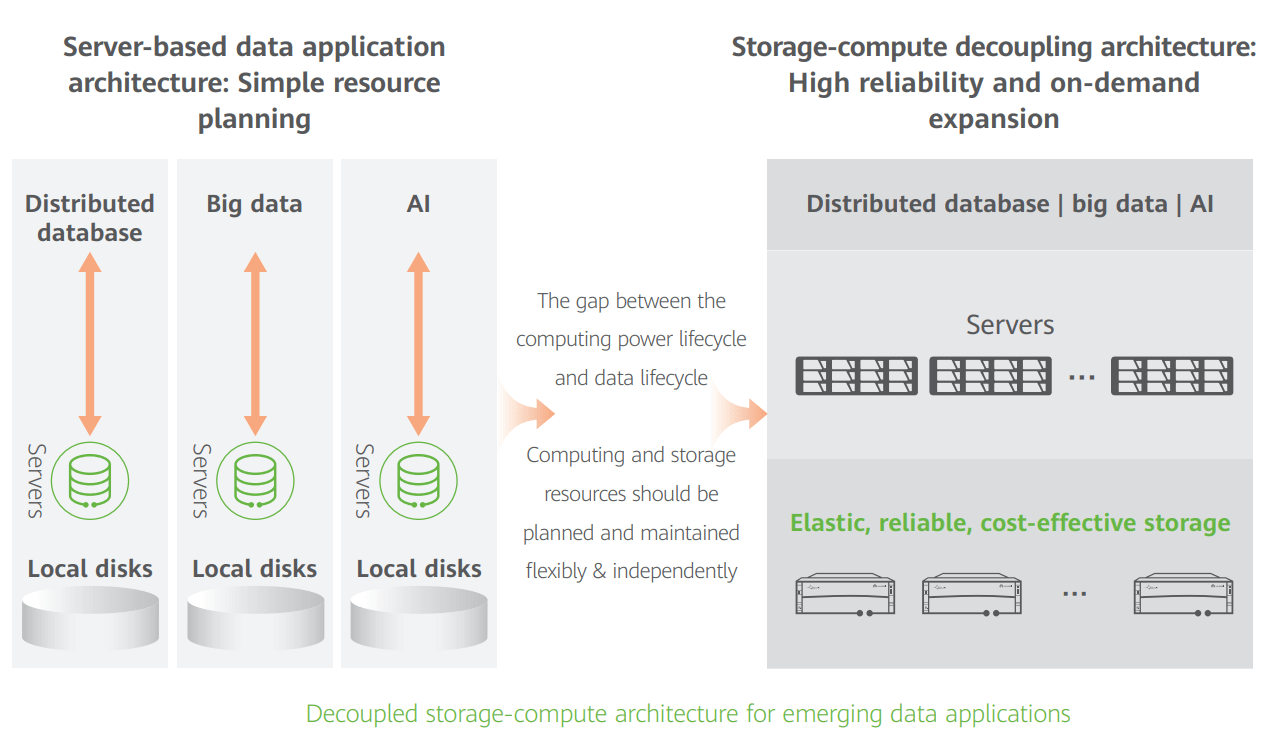
- Deploy IT stacks with acceleration engines to better support diverse data applications
To facilitate diverse data applications, future storage will no longer be just a persistence data carrier. Instead, it will form data infrastructure that combines the persistence layer and data acceleration engines.
The acceleration engines connect the storage layer to different applications for near-data processing, improving processing efficiency by several times and significantly accelerating applications.
Learn more about Huawei’s Data Storage solutions.
Disclaimer: Any views and/or opinions expressed in this post by individual authors or contributors are their personal views and/or opinions and do not necessarily reflect the views and/or opinions of Huawei Technologies.
Leave a Comment