Scale-Out Storage: The Right Choice to Underpin Your AI Solutions
Since its launch in November 2022, ChatGPT, developed by OpenAI, has captivated consumers worldwide and captured the imagination of organizations in various industries.
In 2023, people have further witnessed how generative AI can significantly transform the ways we live and work.
AI is no longer just a buzzword. Instead, organizations can now truly benefit from generative AI in many ways, including content creation, data analytics, customer services, and project O&M — to name just a few.
That's why many enterprises are either preparing to develop or have already started developing their large AI models.
However, have you considered whether your storage can handle the upcoming challenges in the AI era with ease?
Challenges
Data determines the power of AI. As a home to data, storage has become a critical part of infrastructure for large AI models. To fully unleash the potential of AI, storage must overcome the following challenges:
Ever-increasing data volume
Large AI models require huge amounts of data for training, resulting in a significant increase in storage capacity needs. Storing and managing the massive amounts of data generated by AI models is quite challenging.
Data accessibility and availability
Large AI models need fast and reliable access to data during training and inference. Ensuring that relevant and up-to-date data is readily available and accessible is essential for the successful implementation of generative AI solutions.
Performance and scalability
Large AI models can generate and process data at a high rate, requiring storage systems that can scale and perform efficiently to handle heavy workloads. Ensuring that the storage infrastructure can handle the increasing demands of generative AI models is essential.
Data archiving and management
Large AI models generate a large volume of data, which needs to be organized, stored, and managed effectively. Training data may need long-term archiving for future reference or model retraining. Implementing effective data tiering and lifecycle management strategies is necessary but also challenging, especially when dealing with large-scale AI projects.
Designing the ideal storage solution
To address these challenges, the architecture for a storage solution more suited to large AI models needs to have the following features:
1. Multiple tiers with a unified namespace: A single storage system with both a high-performance tier and a large-capacity tier, a unified namespace, and the ability to manage data throughout its entire lifecycle.
First, the storage system needs to allow users to set a placement policy for newly written data. For example, at the data acquisition stage, if newly obtained data needs to be processed immediately, then it would be stored in the high-performance tier. New data that doesn't need to be processed in the immediate future, or data that is used for long-term archiving, would be directly written to the large-capacity tier.
Second, the storage system would have to allow for a flexible mix of data tiering and mobility policies. Data should be allowed to automatically move between tiers based on user-defined policies.
2. Support for all services: A single storage system that can handle all services in the entire AI process. This also includes support for all protocols used by different toolchains throughout the end-to-end development process. It would have to do so without semantic loss, meeting the same compatibility requirements as native protocols.
3. Efficient migration: The storage system needs to support efficient data transfer for seamless collaboration between all stages of the process. Different stages should be able to collaborate with zero data copying and zero format conversion, so the output of the previous stage can be directly used as input for the next stage.
4. Superb scalability: The storage system needs to be able to scale out horizontally to thousands of nodes. It should adopt a fully symmetric architecture without additional metadata service nodes, allowing system bandwidth and metadata access capabilities to increase linearly as new nodes are added.
5. High performance for hybrid workloads: A single storage system that delivers high performance when handling dynamic hybrid workloads.
- At the data ingestion stage, large and small files can be written at the same time.
- At the data preprocessing stage, large and small files can be read and processed in batches to generate massive amounts of small files.
- At the model training stage, massive amounts of small files can be randomly read in batches.
- When generating checkpoints, large files can be written with high bandwidth.
- At the model deployment stage, the same model file can be read with high concurrency and high bandwidth.
To deliver an excellent performance under all I/O models, the ideal storage system for developing AI systems needs solid technical strengths of scale-out storage.
Introducing OceanStor Pacific
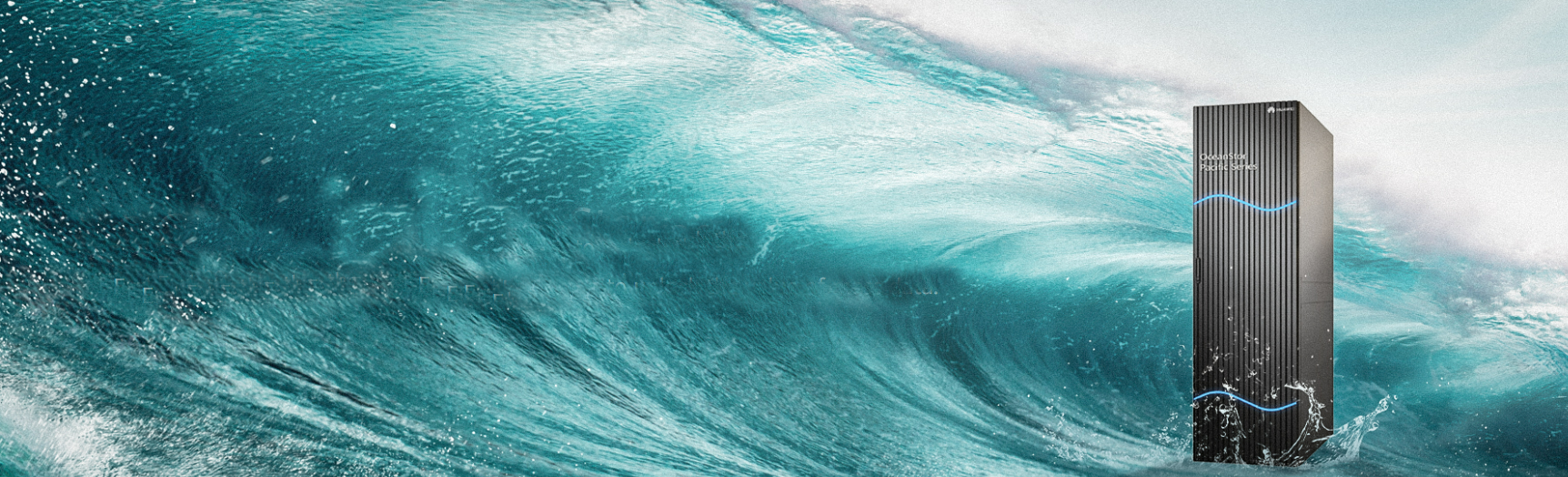
OceanStor Pacific scale-out storage developed by Huawei is the perfect match for AI scenarios, meeting all data storage requirements throughout the entire AI model development process.
- OceanStor Pacific scale-out storage uses dedicated high-performance hardware and a high-density design. It comes packed with a 1:1 bandwidth oversubscription ratio between network and SSD, transparent backup power technology, and I/O passthrough to ensure that performance density is 60% higher than competing products in the industry.
- Its FlashLink technology is designed for flash native. With global garbage collection that can sense the layout of data, intelligent aggregation of data with similar lifecycles, and disk-controller collaboration, FlashLink doubles the service life of SSDs and delivers stable, sub-millisecond latency.
- OceanStor Pacific scale-out storage works with high-performance distributed parallel clients. Different from the standard NFS protocol, where one protocol client is connected to only a single storage node, OceanStor Pacific enables a parallel client to connect to multiple storage nodes at the same time. Even when accessing a single file, concurrent read and write operations are performed on multiple storage nodes. With techniques implemented on the client side – such as data layout sensing, learned index, data passthrough access, and remote direct memory access (RDMA) communication – a single cluster can provide TB-level read/write bandwidth and hundreds of millions of IOPS.
Summary
When it comes to developing AI models, Huawei OceanStor Pacific scale-out storage can help simplify and expedite the process, saving you time, money, and the headache of manually migrating massive amounts of data between disparate systems.
Huawei data storage will continue to innovate and build a new data paradigm to unleash the power of AI.
Learn how you can build sustainable growth for your business with Huawei OceanStor Pacific scale-out storage.
Disclaimer: Any views and/or opinions expressed in this post by individual authors or contributors are their personal views and/or opinions and do not necessarily reflect the views and/or opinions of Huawei Technologies.
Leave a Comment