Don't Let Data Hold You Back: Understanding AI-Ready Data Infrastructure
As mentioned in the previous article, AI-ready data infrastructure has transformative power for all industries.
Today, let's have a look at what exactly AI-ready data infrastructure is and the major characteristics it needs to have to address challenges in the AI era.
What is "AI-Ready Data Infrastructure"?
AI-ready data infrastructure refers to data storage software and hardware systems designed for AI applications and services.
This infrastructure offers large-scale data ingestion and preprocessing, high performance and consistency, and superb resilience to enable AI compute clusters to efficiently analyze data and learn.
Must-have features to turbocharge your AI
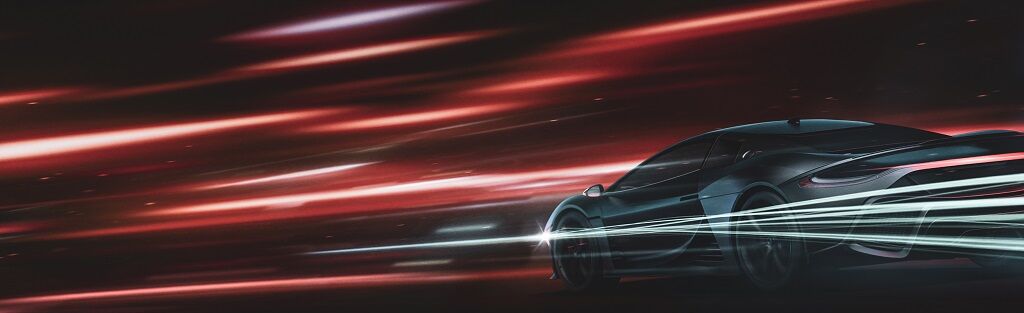
To keep up with AI trends, many organizations are stacking computing power for performance gains, which leads to ever-increasing computing concurrency. This in turn requires larger datasets to be quickly loaded into compute clusters for faster deep learning, which ultimately enhances the capabilities of large AI models.
Therefore, building AI-ready data infrastructure requires comprehensive preparation to ensure that it offers:
- Large-scale data ingestion and preprocessing
- High performance and consistency
- Superb resilience
- Data intrinsic resilience
Today, let's dive into the first two of them.
1. Large-scale data ingestion and preprocessing
Many enterprises store their data in different data centers or on different storage devices in the same data center. Service O&M personnel know what data they have, but do not care where the data is stored. IT O&M personnel know where and how much data is stored, but do not care what the data is.
As a result, enterprises lack a unified view for managing scattered data, let alone carrying out effective data ingestion and preprocessing to support the use of AI computing power for training.
Therefore, AI-ready data infrastructure must enable data owners to perform large-scale data ingestion and preprocessing.
- Ingestion of dispersed data from multiple sources
Using a unified namespace can enable visualized data asset management and policy-based data ingestion from multiple sources and different locations. This approach ensures data visibility, manageability, and availability, which facilitates efficient data access via GPUs/NPUs.
- Data preprocessing
To ensure that there are high-quality datasets for training, consider adopting a framework that simplifies data cleansing, conversion, and standardization.
2. High performance and consistency
In the model training phase, the following processes are greatly influenced by data infrastructure:
- Loading training datasets
- Reading and writing checkpoints
These processes are critical to computing power utilization. As the GPU/NPU quantity in an AI cluster increases from tens of thousands to hundreds of thousands, storage performance needs to keep up to ensure these processes are executed smoothly and efficiently.
- Loading training datasets mainly involves accessing massive amounts of small files. This requires a performance density of million-level OPS per PB to minimize the loading time.
- Reading and writing checkpoints are bandwidth-intensive processes. They require a performance density of TB/s bandwidth per PB to minimize the fault recovery time for a compute cluster.
The performance density must be achieved in both low- and high-capacity scenarios (for example, tens of or even hundreds of PB-level storage capacity) to meet the performance needs of ever-growing compute clusters. This requires data infrastructure to have strong scale-out capabilities for non-disruptive expansion and a near-linear increase in both performance and capacity.
In addition to high performance, the strong consistency of checkpoints written by a compute cluster to the storage is critical. If a compute cluster fails, the latest checkpoint[N] needs to be read to resume training. However, solutions like distributed caching cannot ensure strong consistency of checkpoint data. To resume training in these cases, a compute cluster has to revert to:
The latest complete and available checkpoint[N – x] (where x is an integer ≥ 1).
This renders all training after checkpoint[N – x] invalid, which wastes time and resources.
During data loading or recovery, GPUs/NPUs remain idle. This reduces AI cluster utilization, resulting in a massive waste of resources. Analysis shows that proper storage performance improvement (not just capacity expansion) can greatly reduce the GPU/NPU idle time caused by training dataset loading and checkpoint reads and writes. This can improve cluster utilization by about 10%.
The absolute value of FLOPS can be increased either by improving the performance and consistency of data infrastructure or by stacking computing power, but the former approach is greener, more efficient, and twice as cost-effective.
Summary
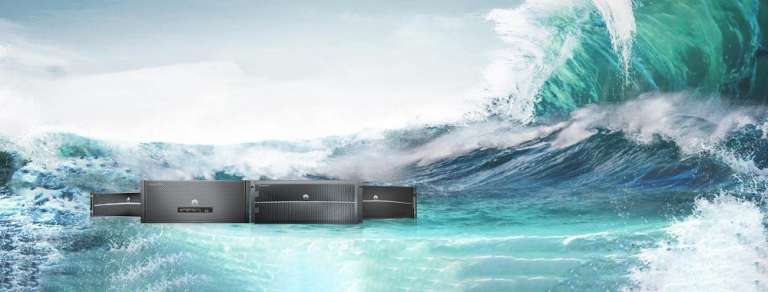
Now, we know what AI-ready data infrastructure is and the major characteristics it should have to help you address challenges in the AI era.
In the next post, we will look at Brilliance in Resilience: How AI-Ready Infrastructure Is Shaping Tomorrow's World.
Huawei is an industry leader with over 20 years of extensive investment in data infrastructure. It offers a broad range of products, solutions, and case studies to help you handle AI workloads with ease. Learn more out our award-winning OceanStor Data Storage and how to unleash the full potential of your data.
Disclaimer: Any views and/or opinions expressed in this post by individual authors or contributors are their personal views and/or opinions and do not necessarily reflect the views and/or opinions of Huawei Technologies.
Leave a Comment